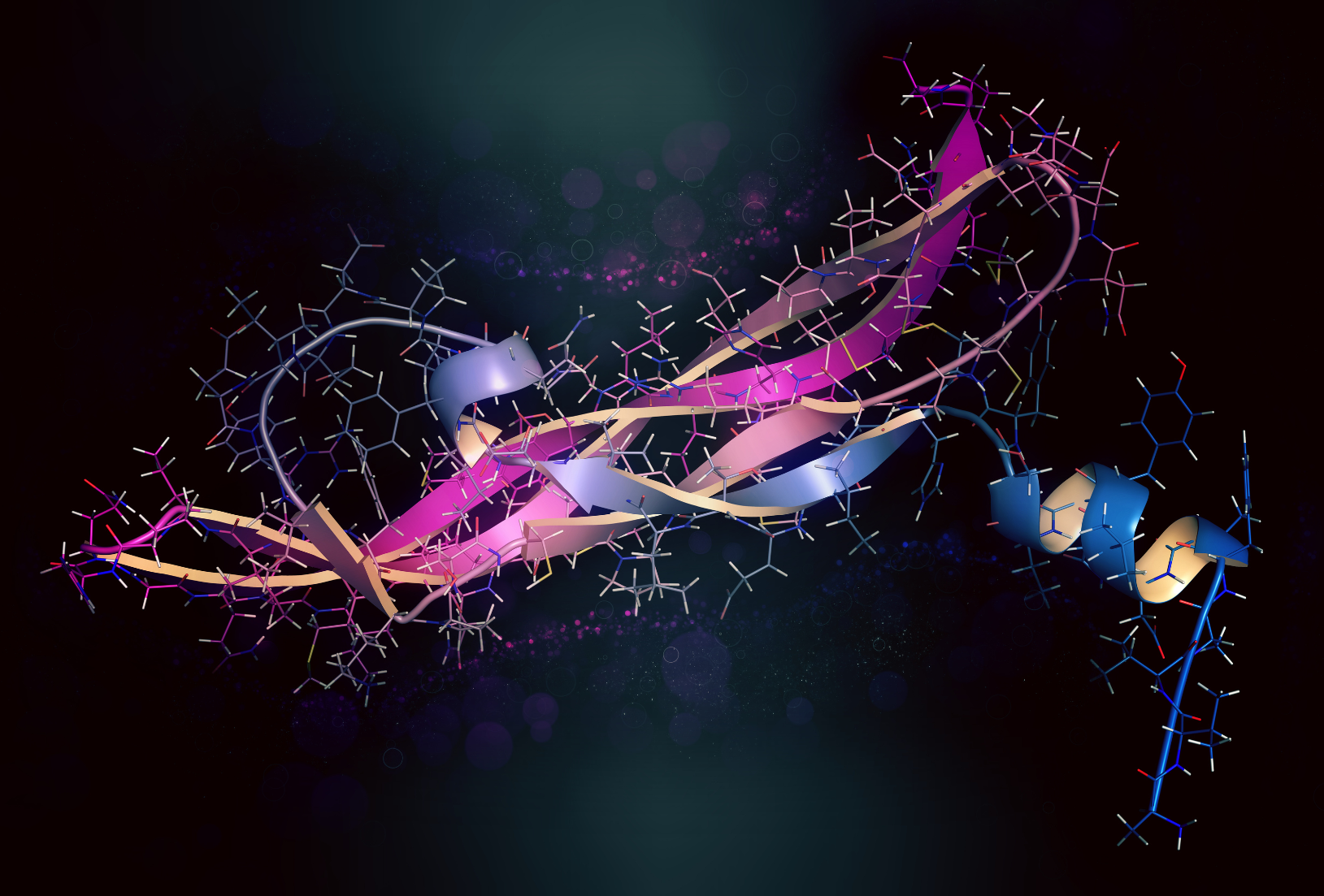
30 May The right tools for the job: How can regulation adapt to the future of drug discovery and development?
In September 1985, Microsoft Excel was released, and the world changed.
Excel was not the first spreadsheet software, but it was the first to implement “intelligent cell recomputation”, whereby changes to one cell automatically resulted in all linked cells (and no others) being updated. With the rise of Excel, tasks which once took hours were suddenly the work of a moment, and it is no surprise that Excel has since become a ubiquitous tool across everything from finance to event management.
Today, we are on the brink of another leap forward.
Consider proteins. The complex, three-dimensional structures of proteins are fundamental to biological processes, yet determining these structures experimentally is an incredibly complex, time-consuming and costly process. Computer modelling offers an alternative, but this can require very large amounts of processing power. This month, however, UK AI leader DeepMind launched AlphaFold 3, offering the potential to predict the structure of proteins (as well as DNA and RNA) in mere seconds. What’s more, DeepMind has made AlphaFold 3 freely available to the public for non-commercial use.
The potential of AlphaFold 3 and other AI and deep learning tools is huge, potentially unlocking whole new areas of personalised medicine.
But with profound advances come profound challenges.
It has been estimated that 88% of Excel spreadsheets contain errors, with (for example) gene names sometimes being autocorrected to dates. These problems were the result of human misuse of a tool. But at least Excel errors are identifiable. The same cannot be said of black box AI tools, particularly those built on deep learning. Yet it is essential that patients and the public are able to trust the products which AI tools help develop. We are already seeing waning public trust in vaccines. If the newly AI-empowered pharmaceuticals sector is to avoid the same fate, effective, trusted regulation is of fundamental importance.
So how can regulation keep pace with this evolving landscape and manage the inherent risk of these new tools? There are no easy answers to this question, but I think a few key principles can help pave a road to success.
First, innovators should engage with regulators early. Bringing regulators along on the journey will give them a better understanding of how AI tools are being used, what the risks are, and how those risks are being mitigated.
In turn, regulators should recognise that, at least for the time being, each AI tool is just that – a tool to be used as part of a larger process of drug discovery. Research carried out in silico will be combined with in vivo and in vitro work to form a coherent whole, and of course double-blinded, randomised control trials will remain the gold standard for assessing the efficacy of a new drug.
Above all, it is essential that regulators and innovators work together to adapt to this changing landscape. Effective regulation of such complex technology is dependent on the expertise of innovators, while innovators in turn rely on regulators to ensure their products are trusted by consumers. If the relationship between regulators and innovators is anything other than collaborative and open, the whole process will break down.
In drug development, as in life, there will always be risks, whether from Excel or AI. The point of regulation, ultimately, is to manage those risks. After all the development of new, safe and effective drugs is something we can all get behind.